In information theory, uncertainty is a physical quantity that characterizes how reliable a random variable is, which is calculated by entropy generally. Today, many machine learning algorithms can map high-dimensional space data into low-dimensional arrays, but the accuracy of these mappings is often overlooked, leading to many catastrophic consequences. Therefore, it is necessary to evaluated the uncertainty of these information.
Existing methods for describing uncertainty include particle filter, conditional random field, etc. But in deep learning, it is often difficult to describe uncertainty. For example, in classification problems, deep learning algorithms often give a normalized confidence vector, but there is hardly a way to get the uncertainty of the model. Uncertainty analysis is one of the main research directions of our laboratory. It aims to use the Bayesian deep learning framework to decide the uncertainty in classification and regression problems.
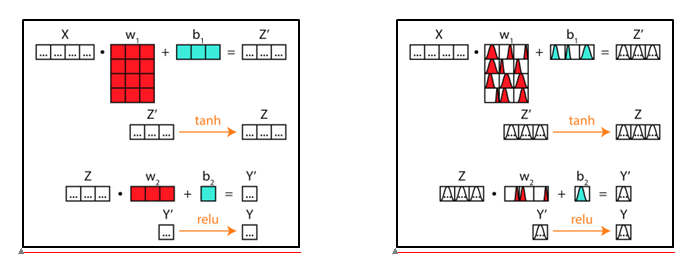